Our data driven approach to leakage
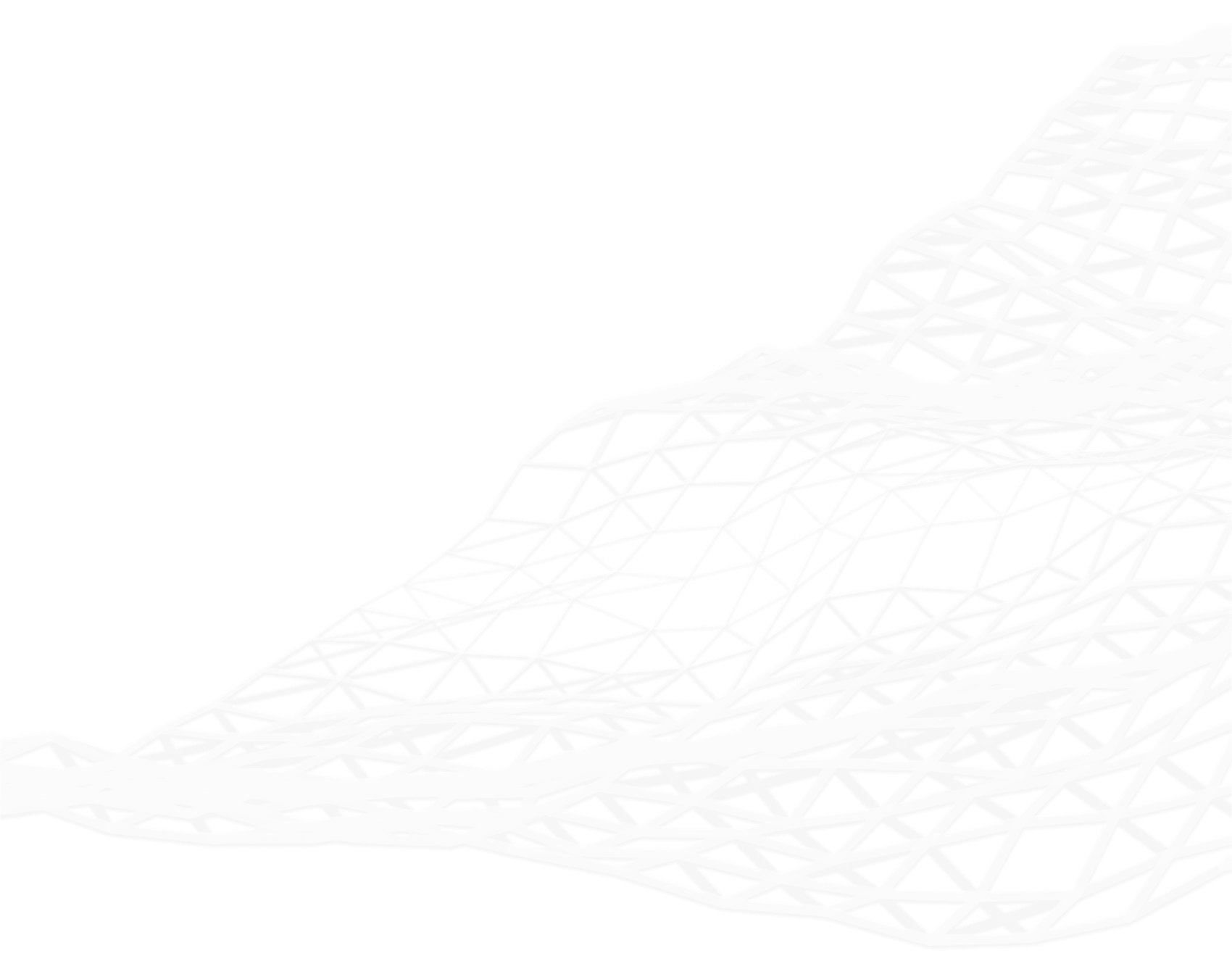
Our data driven approach to leakage
Mastering the management of location data is critical when inferring the location of sewers, migrating to a utility network model, or predicting and preventing leakage. Leakage has been a focus for us here at 1Spatial and we have been innovating and collaborating with water companies on the roadmap for our leakage solution.
We recently spoke at Utilities Week Live in May on “Using advances and innovations in technology to address geographical factors impacting leakage”. In early June, we invited water companies to join us on a webinar for our leakage solution, to review where it is and where we’d like to take it. Jeremy Heath, Innovation Manager at SES Water and UKWIR’s leakage lead, set the scene for us with a summary of the recent leakage report. And we’ve also been speaking to water companies about our solution, and how we can collaborate and innovate to take it forward.
From these conversations is clear there’s no “one solution” for leakage, however, we think our machine learning derived heat map forms a piece of the jigsaw puzzle to help meet NIC and PIC targets.
Our current leakage solution
It’s difficult to effectively detect leaks when using traditional data assessment methods. Our starting point is looking at insights from data available to you. For example, from sensors, existing attributes, terrain and 3rd party datasets; like traffic and weather etc.
Our solution allows you to quickly identify the location of assets that are most at risk of leakage by recognising patterns not otherwise identified by traditional methods and tools, and to make sense of the proliferation of data, making it much easier to integrate more data sets for use in analytical assessments. But what is key here is the data, which sits at the heart of any organisation.
A lot of data is required to train our model and we have to ensure (through our patented rules engine) that this data is clean, correct and unbiased, so we don’t teach the model the wrong thing. Our solution can be used to verify data, clean it up, merge and combine it with different sources and ensure that it is consistent, compliant and complete enough for learning from. Rules based systems can be used to encode this geospatial information into numbers used by the ML processes such as size, shape, proximity, or other geospatial interactions with the surrounding data. Neuro-Symbolic AI acknowledge that rules and machine learning combined are more powerful, as it allows the guiding logic of a person explicitly defining rules alongside the fuzzy inference benefits of machine learning from lots of data.
By QA’ing the data inputs, the key is to be able to develop a system that learns and understands the unobservable and hidden relations between various structural properties of the network, such as the pipe material, along with other parameters that render a pipe vulnerable to leakage such as its age, previous history of leaks either for a similar pipe material or its location, length, diameter or elevation of the install.
By combining rules and Machine learning algorithms across spatial, semi-spatial and non-spatial feature engineering techniques, we create spatially conscious machine learning models which outperform non-spatial models when combined with advanced prediction techniques.
Currently our solution produces a pipe distribution network classified into High, Medium and Low burst propensity to better predict assets that are most likely to fail and result in product losses through leakage.
On the image below we can see the weighted totals of scores that are used to represent the risk of leakage for pipes to help you identify a prioritised list of high-risk leakage areas which are visualised on a "heat map".
Where next?
We started out in 2018 at Northumbria Water simply using our rules engine to produce a heat map for their Essex area that they still use in their GIS today. In 2020 we combined a machine learning approach into our solution and did a hackathon on consumption patterns with Anglian Water. So where next for us?
We’re looking at incorporating real time data from sensors and loggers or smart meter data into the model. Perhaps look at consumption patterns or predicting supply pipes by inference. Can we do inference or modelling of student populations or the changing consumption patterns with the new hybrid working since Covid 19? All of these are possible with data, our rules engine, and machine learning. We're a company that's interested in innovating with data and technology and collaborating with the industry’s views!
Also, think about the possibilities of clean, validated data that can help with sewer inference, network modelling and the building of your digital twin.
It’s all about the Data: Ethics and Governance
Responsible use of data and data ethics has been a hot topic for a number of years and it’s no surprise with the increased use of data that there is increased focus on this
The Geospatial Commission recently published paper, ‘Building Public Confidence in Location Data: The ABC of Ethical Use’. ABC stands for:
- Accountability - Governing location data responsibly, with the appropriate oversight and security
- Bias - Considering and mitigating different types of bias, and highlighting the positive benefits of location data
- Clarity - Being clear about how location data will be used and the rights of individuals
These are the building blocks for good location data governance. They aim to maximise public trust and confidence in the use, sharing and reuse of location data.
The report also identifies three location data trends where ethical considerations apply:
- Real time data is increasingly available thanks to high levels of connectivity as well as the ability to store data in the cloud and access it through edge-computing.
- The proliferation of sensors matching the proliferation of devices that can house these sensors, and decreases in the cost and size of geospatial technology.
- Artificial Intelligence and machine learning is increasingly being used in the geospatial sector to support the processing of vast amounts of data.
Location data is seen as a significant part of this data explosion, particularly as AI and other techniques can quickly join the dots between location and personal data.
Our Location Master Data Management approach draws on our patented rules-based technology, combined with deep data management expertise, to unlock the value of location data. Rules can be used to test assumptions on data, so you can manage the risk effectively and save time and money in doing so. We are often involved in the audit of the data flow, cleaning, integrating and transforming data. We adopt standards in the work that we do, to protect privacy and ensure cyber security while helping to trace decisions back to the data source.
Think about the possibilities of clean, validated data that can help with sewer inference, network modelling and the building of your digital twin. Or even if you are thinking through how you approach migrating to new data models, such as the ArcGIS Utility Network Model. All the above automated and AI techniques can help you confidently migrate to these next-generation systems while keeping data ethics at the forefront.
Get in touch
For more information about our leakage solution, or if you're interested in innovating with us.
Contact Us